- What is Generative AI?
- How Does Generative AI Work?
- The Types of Generative AI Tools
- Core Technologies and Concepts Driving Generative AI
- Benefits of Generative AI
- Limitations, Challenges, and Risks of Generative AI
- How Can Generative AI Drive Business Growth and Value?
- Looking at the Use Cases of Generative AI
- Real-World Case Studies: How Top Companies Are Leveraging Generative AI
- Generative AI in Compliance: Transforming Regulatory Adherence with Intelligent Automation
- Looking at the Future of Generative AI
- Wrapping Up
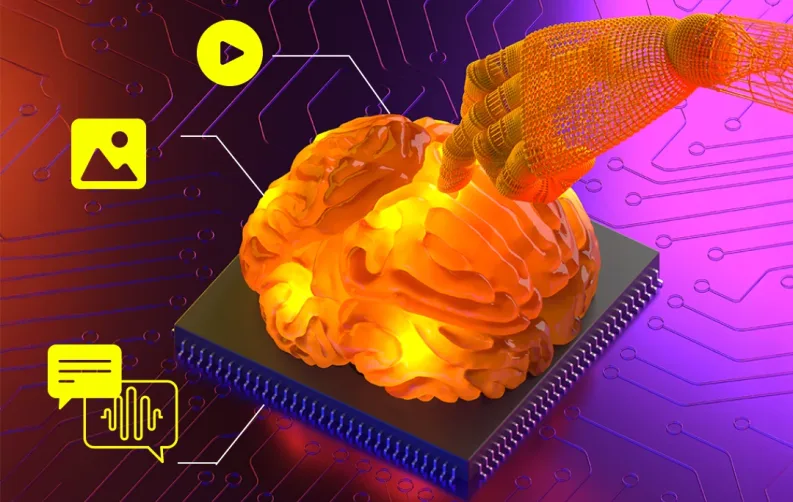
Is Generative AI the Future or Just Another Tech Trend?
Imagine a world where content—from articles to artwork—is created not by humans but by machines. That world is already here, thanks to generative artificial intelligence. This revolutionary technology isn’t just making waves—it’s transforming industries, automating tasks, and redefining creativity. But what is generative AI, and why is it such a game-changer? And why are there debates about Generative AI vs traditional AI?
Unlike traditional AI, which analyzes and classifies data, generative AI creates entirely new content by learning from vast datasets. From chatbots that mimic human conversation to AI-generated music and art, its capabilities seem limitless. McKinsey estimates that generative AI could add up to $4.4 trillion annually to the global economy—proof that it’s more than just hype.
As businesses rush to integrate this technology, ethical concerns and risks emerge. Will it enhance human creativity or replace it? This article dives deep into what generative AI is, how it works, and the impact it’s set to have on our world.
What is Generative AI?
Generative AI, commonly referred to as gen AI, is artificial intelligence (AI) that can produce unique material in response to a user's prompt or request. This includes text, photos, video, audio, and software code.
Advanced machine learning models known as deep learning models—algorithms that mimic the learning and decision-making processes of the human brain—are the foundation of generative AI. By finding and encoding patterns and relationships in vast volumes of data, these models are able to comprehend users' natural language queries and requests and provide pertinent new content in response.
Although artificial intelligence (AI) has been a popular technology issue for the previous ten years, generative AI—more especially, ChatGPT's introduction in 2022—has made AI the focus of international attention and sparked an unprecedented wave of AI adoption and innovation. Businesses are moving forward, investigating how generative AI may enhance their internal workflows and improve their goods and services despite the fact that it also poses very real risks and obstacles. Generative AI offers tremendous productivity gains for both individuals and organizations. A third of companies are currently routinely utilizing generative AI in at least one business function, per a study conducted by the management consulting firm McKinsey.
By 2026, over 80% of businesses will have implemented generative AI applications or utilized generative AI application programming interfaces (APIs), according to industry analyst Gartner.
How Does Generative AI Work?
Generative AI models use neural networks to find patterns and structures in existing data in order to produce unique and fresh material.
One of the innovations of generative AI models is their capacity to use various learning strategies, such as semi-supervised or unsupervised learning, for training. As a result, businesses may now more readily and rapidly use a lot of unlabeled data to build foundation models. Foundation models, as their name implies, can serve as the basis for multitasking AI systems.
GPT-3 and Stable Diffusion are two examples of foundation models that let users take advantage of the language's capabilities. For instance, well-known programs like ChatGPT, which is based on GPT-3, let users create essays in response to brief text requests. However, with Stable Diffusion, users can use text input to create photorealistic visuals.
Ever Wondered: How is Generative AI trained? Answer: Generative AI is trained using vast datasets and advanced machine learning models. It learns patterns, structures, and relationships through deep learning techniques like neural networks. By processing massive amounts of data, it refines its ability to generate text, images, and more. The result? AI that mimics human creativity with stunning accuracy! |
The Types of Generative AI Tools
With advancements in types of artificial intelligence, various generative AI tools have emerged, each serving unique purposes across industries. From text generation to image creation and beyond, these tools leverage deep learning to produce human-like content. But what are generative AI tools, and how do they differ? Understanding their types helps businesses and individuals harness their potential effectively.
1. DALL·E 3
With good cause, DALL·E 3 is at the forefront of AI image production. For starters, it constantly creates remarkably detailed and lifelike images. Another is that it's quite easy to use. All you need to do is prompt ChatGPT or Bing like you normally would, and you'll have two to four AI-generated options to pick from in a matter of seconds because it's accessible through ChatGPT, Microsoft Copilot, and a few other services that use its API. Microsoft offers significantly more versatility without charging, while OpenAI allows ChatGPT members to produce two photos each day with DALL·E 3.
2. ChatGPT
The most well-known and original of its kind, ChatGPT, swiftly ascended to the top of the AI chatbot food chain. It has evolved from a party trick to a truly practical tool since it first dominated dinner party conversations everywhere. Its adaptability is unparalleled, particularly with the introduction of more potent multimodal models like the o1 series and GPT-4o. ChatGPT can write code, understand audio, translate across languages, answer questions, and search the web. Using GPTs, you may even create your own personalized bot with distinct data and instructions.
3. Adobe Photoshop
Adobe Photoshop, which is widely recognized as the industry standard for picture editing software, was not exempt from the AI effect. Photoshop uses a variety of generative capabilities from Adobe's Sensei and Firefly AI, but it is not just an AI image production tool. With Adobe Firefly, you can quickly eliminate extraneous objects from photos, improve resolutions for sharper images, and create completely new image components. Neural filters are also capable of removing compression artifacts, transferring the color palette between images, and automatically adjusting the facial expression of your subject.
4. Runway
Runway approaches the creation of AI videos in a novel way. The majority of generative AI solutions that work with the video format often use pre-made templates. However, Runway enables you to record and produce own videos in response to instructions. It will be challenging to achieve the precise outcomes you desire, particularly in the beginning, but Runway offers an Academy with a wealth of tutorials and videos to help you understand how the platform operates. Additionally, you can use this prompting guide to help you anticipate the outcomes. The learning curve is less steep, thanks to these materials.
5. Jasper
The multipurpose AI writing assistant Jasper (previously Jarvis) is renowned for its vast features and easy-to-use interface. With this tool, you may create a variety of content, from product descriptions and innovative sales copy to blog entries and social media captions. It focuses on marketing efforts and provides resources for creating blog posts, social media material, and text that converts well. However, at $39 per month (paid annually), it's not the most affordable AI option available, so marketing teams on a limited budget might find it too costly.
Core Technologies and Concepts Driving Generative AI
The operation of generative AI tools is made possible by a number of fundamental ideas and technologies. These are some of the types of generative AI you need to know:
1. Neural Networks and Deep Learning
These are the fundamental components of generative artificial intelligence. Machines can identify patterns and relationships in data thanks to neural networks, which mimic how the human brain processes information.
Neural networks with several layers that are capable of learning hierarchical representations are used in deep learning. This makes it possible to create intricate and subtle information using a variety of data formats, including text, photos, and music.
2. Transformers
Transformers are sophisticated models that aid in comprehending human language context. We refer to this as natural language processing.
The developments in transformers, which allow these AI tools to quickly produce logical and contextually relevant text as answers to human queries, impress many new users of programs like ChatGPT. (Note: generative pre-trained transformer is what ChatGPT's GPT stands for.)
3. Variational Anitencoders (VAEs)
Artists who study a lot of paintings and then produce new works in the same style are known as VAEs. For example, they can create new objects that seem like the originals after learning the substance of the data, such as what makes a Van Gogh identifiable as such. Importantly, the output will differ from the original dataset but be comparable.
4. Generative Adversarial Networks
Consider a discriminator and a generator as two players in a game.
- Based on a dataset, the generator generates fresh data points and fictitious visuals.
- The discriminator compares photos to genuine data in an attempt to identify whether they are real or phony.
The artist becomes more skilled at creating realistic visuals to trick the detector over time. GANs strive to create high-quality photos and videos through this adversarial training process—ai-generated content that might appear authentic.
5. Diffusion Models
Like sculpting a lump of clay into a sculpture piece by piece, these models take random noise and transform it into meaningful data over time. They gradually improve the data till the final product is coherent and clear. As a strong substitute for GANs, diffusion models are gaining popularity because of their remarkable ability to produce high-resolution images.
6. Reinforcement Learning
Consider training a dog by rewarding excellent behavior with treats. In a similar vein, AI models are trained to make better decisions over time by learning the optimal course of action through incentives or penalties. In this manner, AI specialists and engineers can mold the intended functionality of AI models, optimizing them to accomplish increasingly precise objectives.
For instance, while training models, AI engineers can utilize reinforcement learning to reward models that follow ethical standards.
7. Quantum Computing
Super-powerful devices, quantum computers can do intricate computations more quickly than conventional computers. Although it is currently in its early phases of research, quantum computing could significantly improve the capabilities of opening up a new generative AI window by more effectively managing bigger datasets and intricate models.
By pushing the limits of what is generative AI, we can accomplish and reduce environmental costs, which could result in considerable improvements in processing power.
A Curious Question: What Are Generative AI Models That Redefine Creativity or Mimic Intelligence? Answer: Generative AI models aren’t just mimicking intelligence—they’re transforming how content, code, and even art are created. These models analyze vast datasets to generate human-like text, images, and more. While challenges exist, their ability to automate creativity, enhance efficiency, and unlock innovation makes them a game-changer in AI-driven industries. |
Benefits of Generative AI
Three major characteristics of generative AI—knowledge synthesis, human-AI collaboration, and speed—are what make the reasons for what makes generative AI unique. Having one or more of these three characteristics can help businesses reap the benefits more quickly, easily, and efficiently, even though many of the benefits listed below are identical to those previously promised by AI models and automation tools.
Enhanced Productivity
By using generative artificial intelligence, knowledge workers can spend less time on mundane daily tasks like organizing or classifying data, searching the internet for relevant research, writing emails, or learning a new discipline that is unexpectedly required for an impending project. AI integration with Android app development is transforming workflows by streamlining coding processes and improving app performance. Fewer workers can complete jobs that once took big teams or hours of work in a fraction of the time by utilizing generative AI. For instance, a group of programmers could spend hours sifting through faulty code to figure out what went wrong, but a generative AI tool would be able to identify the mistakes quickly and report them along with potential solutions.
Working with a generative AI system can significantly increase the productivity of its human partner since some generative AI models have abilities that are about average or superior across a wide range of knowledge work capabilities. With an AI coach by their side, a junior product manager, for instance, maybe at least a mediocre project manager. Knowledge workers' ability to finish a project would be significantly accelerated by all of these qualities.
Decreased Costs
Due to their speed, generative AI technologies lower the cost of finishing procedures; a task that takes half as long to complete will cost half as much as it would otherwise. Furthermore, generative AI can detect redundancies and other expensive inefficiencies, reduce errors, and decrease downtime. However, there is an offset: Due to generative AI's propensity for hallucinations, human supervision and quality control are still required.
However, it is anticipated that human-AI partnerships would accomplish far more work in less time than humans alone—better and more precisely than AI tools alone—which will save expenses. For instance, generative AI can assist in producing more intricate and sophisticated simulations during product testing than could be achieved using more antiquated techniques. As a result, testing new items takes less time and money.
Increased Customer Satisfaction
With generative AI-based self-service and generative AI tools "whispering in the ear" of customer support representatives, providing them with real-time knowledge, customers can have a better and more customized experience. Based on the quality of today's ChatGPT discussions, it's feasible to envision a far higher quality customer experience powered by a company's carefully trained generative AI model, even though the AI-powered customer service chatbots available today can occasionally feel frustratingly constrained.
Improved decision-making: Through scenario modeling, risk assessment, and other advanced predictive analytics techniques, enterprise-specific generative AI models with specialized training can offer comprehensive insights. Through individualized recommendations and practical strategies based on more comprehensive data and quicker analysis than human analysts or outdated technology could produce on their own, decision-makers can use these tools to better understand their industry and the company's place in it.
Improved Decision-Making
Through scenario modeling, risk assessment, and other advanced predictive analytics techniques, skilled, enterprise-specific generative AI models can offer comprehensive insights. Decision-makers can use these tools to better understand their industry and the company's place in it by using personalized recommendations and actionable strategies that are based on faster analysis and more comprehensive data than could be produced by human analysts or outdated technology alone.
For instance, by combining internal data gathered by their enterprise resource planning (ERP) system with extensive external market research, which is subsequently examined by a specialized generative AI model, decision-makers can more accurately forecast demand and better plan inventory allocation prior to a busy season. Better allocation choices in this situation maximize possible sales while reducing overbuying and stockouts.
Faster Product Launches
Compared to earlier times, generative AI can generate product prototypes and initial drafts much more quickly, assist in improving ongoing projects, and test and troubleshoot ongoing projects to identify improvements.
Quality control
A customized, enterprise-specific, generative AI model is likely to reveal errors and discrepancies in the user guides, films, and other materials that a company makes available to the general public.
Limitations, Challenges, and Risks of Generative AI
Although generative AI has advanced remarkably in a short amount of time, there are still many hazards and difficulties for developers, consumers, and the general public. Some of the most pressing problems are listed below, along with solutions.
"Hallucinations" and Other Erroneous Results
A generative AI output that is completely erroneous or incomprehensible but, much too frequently, appears completely convincing is called an AI hallucination. The most well-known instance is when a lawyer utilized a general artificial intelligence (gen AI) program to conduct research for a well-known case, and the technology "produced" a number of fictitious sample cases, complete with quotes and attributions.
According to some practitioners, hallucinations are an inevitable byproduct of striking a balance between a model's creative potential and accuracy. However, developers can use guardrails—preventive measures—to limit the model to reliable or pertinent data sources. Hallucinations and errors can also be decreased with ongoing assessment and adjustment.
Unreliable Results
The same inputs can produce slightly or dramatically varied outcomes because most AI models are variational or probabilistic. In other applications, like chatbots for customer service, where consistent results are required or preferred, this may not be desirable. Users can create prompts that reliably produce the outcomes they desire from their generative AI applications by iteratively improving or compounding prompts, a process known as prompt engineering.
Prejudice/ Bias
Generative models have the potential to produce biased, unfair, or offensive material by picking up on societal prejudices seen in the training data, labeled data, external data sources, or human assessors who refine the model. Developers must make sure that their training data is diverse, set rules for avoiding bias during training and tuning, and continuously assess model outputs for bias and correctness in order to avoid biased results.
Absence of Metrics and Explainability
Even the engineers or data scientists who develop the underlying algorithm can comprehend or explain what precisely is happening inside it and how it arrives at a particular result. However, many generative AI models are "black box" models, which means that it can be difficult or impossible to understand their decision-making processes. The processes and results of generative models can be better understood and trusted by practitioners and users with the aid of explainable AI methods and methodologies.
It might be difficult to evaluate and contrast the quality of generated content. The subtle facets of creativity, coherence, and relevance might not be well captured by conventional evaluation tools. Research on creating solid and trustworthy evaluation techniques for generative AI is still ongoing.
Risks to Intellectual Property, Privacy, and Security
By creating convincing phishing emails, phony identities, or other harmful information, generative AI models can be used to trick users into doing activities that jeopardize security and privacy. Users and developers must take care to ensure that any data entered into the model (during tuning or as part of a prompt) does not reveal any information that is protected by other organizations' intellectual property (IP) or their own. Additionally, they must keep an eye out for fresh content that compromises the intellectual property of others or exposes their own.
Deepfakes
Deepfakes are photos, videos, or sounds that have been artificial intelligence (AI) generated or altered to give the impression that someone is doing or saying something they haven't. They are some of the most horrifying illustrations of how generative AI's potential might be used maliciously.
Most people are aware of deepfakes, which are made to propagate false information or harm people's reputations. Deepfakes have recently been used by hackers in financial fraud schemes and cyberattacks (such as voice phishing scams).
Scientists are working hard to develop generative AI algorithms that can more accurately identify deepfakes. The harm that deepfakes can cause can be mitigated in the meantime by user education and recommended practices (such as refraining from spreading disputed material that has not been verified or approved).
Here’s a Thought: Can Generative AI Ever Be Completely Reliable? Answer: Not quite! While generative AI has made incredible strides, it still struggles with accuracy, bias, and unpredictable outputs. Issues like hallucinations, deepfakes, and intellectual property risks highlight the need for continuous improvements. Developers are working on solutions, but complete reliability remains a challenge. For now, businesses must use AI cautiously, implementing guardrails and human oversight to minimize errors and maximize benefits. |
How Can Generative AI Drive Business Growth and Value?
New and innovative options to boost income, save expenses, boost productivity, and better manage risk are presented by generative AI. It will soon become a differentiator and competitive advantage in the future of AI.
The opportunities are divided into three groups as per Gartner analysis.
Revenue Opportunities
- Product development: Businesses will be able to produce new goods faster thanks to generative AI. Novel flavors and perfumes, new alloys, less hazardous home cleaners, new medications, and quicker and more accurate diagnostics are a few examples.
- New revenue streams: According to Gartner research, businesses with higher AI maturity levels will see higher revenue gains.
Productivity and Cost Opportunities
- Augmenting workers: Generative AI can help workers create and modify text, photos, and other types of material. Additionally, it can create, translate, and validate software code, classify, summarize, and simplify material, and enhance chatbot functionality. At this point, the technology is very good at producing a variety of artifacts fast and in large quantities.
- Long-term talent optimization: Workers' capacity to develop, implement, and improve concepts, initiatives, procedures, services, and connections in collaboration with AI will set them apart. This mutually beneficial interaction will significantly increase the range and competency of workers overall and speed up the time to proficiency.
- Process improvement: Large content repositories that may have been mostly untapped up until now can yield genuine, in-context value thanks to generative AI. Workflows will be altered by this.
Risk Opportunities
- Risk reduction: By analyzing and offering a deeper and wider view of data, including consumer transactions and possibly flawed software code, generative AI improves pattern detection and speeds up the process of identifying any dangers to the company.
- Sustainability: By integrating sustainability into decision-making, product design, and processes, generative AI may assist businesses in meeting sustainability standards and reducing the risk of stranded assets.
As said by Mr. Anup Nair, CTO of Mphasis.AI, in an interview with MobileAppdaily:
The objective of generative AI is the generation of relevant, brand-new, original content based on the learnings from the patterns of data that you provide. It is primarily focused on content generation instead of traditional models that primarily work on prediction and classification problems. It can have human-like conversations where you can have Q&A as a human, and it will generate content for you. So that’s generative AI in its simplest layman form.
To know more about how Generative AI can fuel businesses, dive into this exclusive interview and get to know his perspective.
Looking at the Use Cases of Generative AI
A few examples of generative AI use cases for businesses are listed below. We can anticipate seeing many more as technology advances and businesses incorporate these tools into their operations.
The User Experience
By leveraging smart AI technologies to write material for blogs, web pages, collateral, emails, and more, marketing businesses can increase the output of content while saving time. However, depending on the time, location, and target audience of the advertisement, generative AI systems can also instantly create highly customized marketing copy and images.
Additionally, it will power next-generation chatbots and virtual agents that can respond to customers in a tailored way and even take action on their behalf. This is a big improvement over the earlier generation of conversational AI models that were trained on a smaller amount of data for very particular tasks.
Modernizing Applications and Developing Software
Writing new code can be sped up and automated with code generation tools. By automating a large portion of the repetitious coding needed to update legacy apps for hybrid cloud settings, code generation also holds the potential to significantly speed up application modernization.
Digital Work
Contracts, invoices, bills, and other digital or physical "paperwork" can be swiftly created or revised by generative AI, freeing up staff members to work on more complex projects. Workflows in almost every corporate department, including finance, legal, procurement, and human resources, can be sped up by this.
Research, Engineering, and Science
Models of generative AI can assist engineers and scientists in coming up with original answers to challenging issues. For instance, generative models can be used in the healthcare industry to create synthetic medical pictures for medical imaging system testing and training.
Real-World Case Studies: How Top Companies Are Leveraging Generative AI
Generative AI is transforming industries by automating complex tasks, enhancing user experiences, and unlocking new business opportunities. AI use cases are evident in projects by leading AI development companies, showcasing the true potential of this technology. Below are some of the most innovative Generative AI examples from top firms, highlighting how AI is reshaping various sectors.
Appinventiv: AI-Powered Budget Management Chatbot
Managing finances can be tedious, especially for millennials who prefer interactive and engaging experiences. Appinventiv, an India-based app development company, tackled this challenge for one of its clients, the Mudra budget app, by developing a chatbot-driven budget management app designed to simplify financial tracking with AI-powered automation.
The Solution
The app uses Google Dialogflow to fetch financial data from users' debit and credit card history. It then interacts with them through a chatbot that makes budgeting more engaging using puns and gamified elements. The user-centric design ensures a seamless and intuitive experience, making financial planning more accessible.
Results Delivered
- Automated expense tracking for hands-free budget management
- Interactive AI chatbot for real-time financial insights
- Engagement-focused UI/UX tailored for millennials
- Launch-ready across 12+ countries, with plans for global expansion
Codewave: Turning Static Images into Interactive Assets
What if every image on the internet could be interactive and generate revenue? Codewave, a design-thinking-led digital transformation company, developed a Generative AI-based image transformation tool that revolutionizes how businesses and users interact with digital images.
The Solution
A US-based AI startup partnered with Codewave to develop an advanced machine-learning model capable of recognizing objects, people, and contextual elements within images. This system overlays interactive elements on static images, creating engaging micro-journeys for eCommerce, digital marketing, and online marketplaces.
Results Delivered
- Advanced image recognition for object and context detection
- AI-powered interactive overlays to engage users dynamically
- Usage analytics to track and optimize customer interactions
- Scalable deployment across multiple platforms for enhanced monetization
Addepto: AI-Driven Passenger Assistance Bot
With air travel becoming more complex, Addepto, AI consulting company, partnered with a global leader in airport communication technology to enhance passenger experience using AI-driven assistance bots.
The Solution
The project involved GPT-4-powered AI bots integrated with facial recognition technology to assist both passengers and airport staff. The AI chatbot provided real-time, human-like responses to queries related to airport navigation, security procedures, and boarding information, streamlining the overall travel experience.
Results Delivered
- Seamless passenger assistance with AI-driven responses
- Improved airport operations through automated staff support
- Integration of facial recognition for faster verification
- Cloud-based AI infrastructure for scalability and efficiency
These case studies are just a glimpse of how Generative AI is driving real-world transformation. If you're looking for the right AI development partner, explore our directory of 100+ Generative AI development companies to find the perfect match for your project.
Generative AI in Compliance: Transforming Regulatory Adherence with Intelligent Automation
Generative AI is revolutionizing regulatory compliance by automating processes, enhancing accuracy, and providing real-time insights. As organizations face increasing regulatory complexities, generative AI algorithms offer advanced solutions to streamline compliance tasks, interpret intricate regulations, and improve risk management. From financial monitoring to supply chain compliance, AI-powered tools are reshaping how businesses adhere to legal frameworks.
How Generative AI Tackles Compliance Challenges
Generative AI has emerged as a potential game-changer for compliance, offering several ways to assist compliance professionals.
Adapting to Regulatory Changes
Regulatory landscapes evolve rapidly, making compliance a moving target. Types of generative AI, such as Natural Language Processing (NLP) models, continuously analyze and interpret new laws, ensuring organizations remain compliant without manual intervention.
Interpreting Complex Regulations
Legal jargon can be overwhelming, leading to misinterpretations and compliance errors. AI-driven NLP systems process large volumes of legal texts, providing clear and actionable insights for compliance teams.
Enhancing Compliance Coordination
In multinational organizations, maintaining consistency across jurisdictions is challenging. Generative AI centralizes compliance data, streamlining communication and ensuring seamless adherence to diverse regulatory requirements.
Integration with Legacy Systems
Many businesses struggle with outdated compliance infrastructures. Generative AI bridges the gap by enabling smooth integration, ensuring data consistency, and modernizing compliance workflows.
Supply Chain Monitoring
Tracking supplier compliance can be labor-intensive. AI-powered monitoring tools provide real-time verification, reducing risks and improving transparency across the supply chain.
Customized Training Programs
Ensuring employees understand compliance policies is crucial. Generative AI examples include adaptive training programs that personalize learning modules, improving awareness and reducing unintentional breaches.
Use Case: Generative AI in Financial Compliance
Financial institutions must detect and prevent financial crimes like money laundering. Generative AI generates synthetic datasets to train machine learning models, improving the identification of suspicious activity. Compliance teams can then use these insights to file accurate Suspicious Activity Reports (SARs) and mitigate regulatory risks efficiently.
By leveraging generative AI, businesses enhance compliance accuracy, reduce costs, and maintain regulatory integrity, positioning themselves for a future of intelligent and automated compliance management.
Looking at the Future of Generative AI
It is yet unknown how generative AI will affect companies and human labor. However, one thing is certain: generative AI is receiving enormous investments across a variety of human endeavors.
Generative AI firms are receiving rapid investments from venture capitalists, well-established companies, and almost every other type of business. LLMs' global "magic" is their remarkable capacity to mediate human interaction with massive data and assist individuals in making sense of information by providing clear, concise, and remarkably quick explanations. This implies that generative AI will be incorporated into numerous current applications and lead to the development of a second generation of new ones.
For instance, according to Gartner, by 2024, conversational AI will be integrated into 40% of workplace applications; by 2025, 30% of enterprises will use AI-augmented development and testing methodologies; and by 2026, over 100 million employees will work alongside "robocolleagues."
Over the next five years, generative AI is expected to have a significant impact on businesses. As per Gartner's prediction,
- Conversational AI will be integrated into 40% of enterprise applications by 2024, up from less than 5% in 2020.
- Compared to 5% in 2021, 30% of businesses will have adopted an AI-augmented development and testing strategy by 2025.
- 60% of the design work for new websites and mobile apps will be automated by 2026 thanks to generative design AI.
- More than 100 million people will collaborate with robocolleagues by 2026.
- Almost 15% of new applications will be created automatically by AI without human intervention by 2027. Today, none of this is taking place.
It's difficult to predict the future of generative AI and how it will affect things down the road. However, we will eventually have to reconsider the nature and worth of human expertise as we continue to use these tools to automate and enhance human work.
Wrapping Up
Generative AI is a groundbreaking technology that creates new content, ranging from text and images to code and simulations. It operates using generative AI algorithms, such as GANs and transformers, which learn patterns from vast datasets to generate human-like outputs. Types of generative AI include text-based models like GPT, image generators like DALL·E, and music composition tools. Real-world generative AI examples span industries, from automating content creation to enhancing medical research and financial risk analysis.
Ethical issues and appropriate AI development are still vital as companies and individuals use this technology. Although generative AI fosters creativity and efficiency, maintaining openness and reducing biases are major obstacles. Technology will continue to change sectors as it develops, providing countless opportunities but necessitating close supervision to optimize gains and reduce hazards.

Sr. Content Strategist
Meet Manish Chandra Srivastava, the Strategic Content Architect & Marketing Guru who turns brands into legends. Armed with a Masters in Mass Communication (2015-17), Manish has dazzled giants like Collegedunia, Embibe, and Archies. His work is spotlighted on Hackernoon, Gamasutra, and Elearning Industry.
Beyond the writer’s block, Manish is often found distracted by movies, video games, AI, and other such nerdy stuff. But the point remains, If you need your brand to shine, Manish is who you need.