- The Rise of AI-powered Diagnostics
- How AI Diagnostics are Integrated into Mobile Apps
- Leading AI Diagnostic Tools and Vendors
- Top Use Cases in Healthcare Apps
- Future Trends in AI Diagnostic Tools for Apps
- Challenges and Ethical Considerations for Developers
- Development Costs and Considerations
- Why This Matters for Healthcare App Developers and Tech Professionals
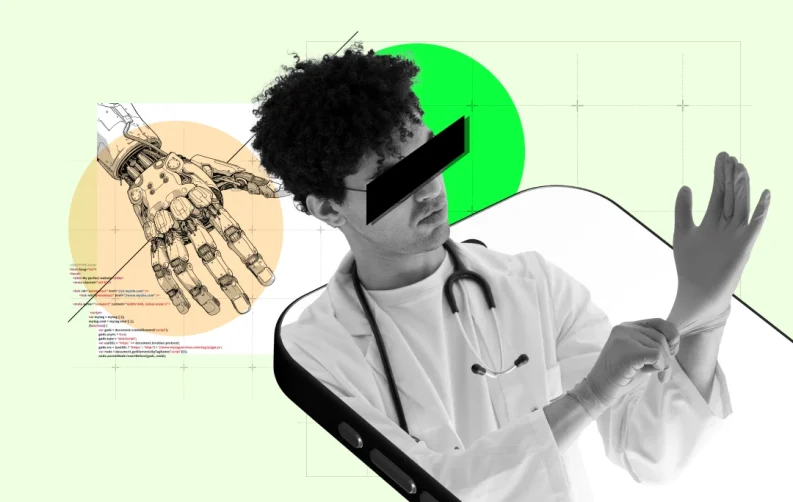
We live in a world today where waking up with a cough or fever doesn’t translate to having to look for an emergency drop-off to the hospital. Instead, cutting-edge examples, like using AI in healthcare diagnostics, can provide near-accurate analysis of health at home. These diagnostics are great for taking care of minor health blips now and then.
Each role of AI in healthcare is powered by machine learning to analyze symptoms, medical data, and patterns, delivering fast, data-driven diagnostic insights.
Apps like Ada and Babylon pioneer reliable AI diagnostics, inspiring developers to boost app accuracy and trust!
But how exactly is AI reshaping healthcare app development?
Let’s dive into the details and explore why this technology is a game-changer for both users and creators.
The Rise of AI-powered Diagnostics
Why are AI diagnostics suddenly everywhere? The answer lies in our growing need for speed and accuracy. Countries like the USA, India, the UK, Australia, etc. are significantly experimenting with the abilities of the tech to find the best AI for medical diagnosis that can change everything. Given the speed at which these countries are innovating, it’s not hard to predict that their healthcare system might soon witness a huge amount of automation.
- In India alone, the market of AI diagnostics in healthcare is rising at a CAGR of 23.17% between 2024 and 2030.
- Companies like Niramai, Predible Health, and Qure.ai are leading this transformation with innovative diagnostic tools.
- People want answers—fast, and this taps into a psychological principle: the need for instant gratification. When you’re worried about your health, waiting isn’t an option.
- AI delivers the solution that is needed, making it the best possible tech for accessible medical diagnosis for millions.
- For instance, AI-powered healthcare apps like Ada Health use AI for health diagnostics to assess symptoms and provide personalized insights.
- These systems don’t just guess—they analyze vast datasets to offer accurate results, often rivaling human doctors.
- This reliability builds trust, a key psychological trigger. When users see that AI diagnostics in healthcare can be as good as (and much faster than) a doctor’s visit, they’re hooked. Of course, it’s always advised to rely on the actual medical staff for a final diagnosis and treatment plan.
How AI Diagnostics are Integrated into Mobile Apps
Well, at the core, AI integration in healthcare apps involves machine learning models trained on vast datasets like millions of medical records, symptom patterns, and outcomes.
These models, often built using frameworks like TensorFlow or PyTorch, are fine-tuned to recognize patterns in user inputs, such as symptoms or vitals, and deliver accurate diagnoses.
Additionally, a symptom checker app might use a natural language processing (NLP) model to parse user inputs (“I have a fever and cough”) and a classification model to map those symptoms to conditions like the flu or COVID-19.
It also integrates convolutional neural networks (CNNs) for image-based diagnostics, such as X-ray analysis. Apps like Symptomate achieve 92% accuracy by blending NLP and deep learning.
Beyond that, apps like Ada Health and Symptomate rely on such pipelines, combining NLP with deep learning to achieve high accuracy in AI diagnostics in healthcare.
For developers, this means an opportunity to build the best AI app for medical diagnosis—one that users trust as much as a doctor’s visit.
For more details, here’s a high-level integration process for healthcare app development powered by AI:
- Data Preparation: Sources can be diverse to train your model. For instance, anonymized medical datasets like MIMIC-III to train your model, ensuring inclusivity to avoid bias.
- Model Training: The process involves using supervised learning to train on labeled data (symptoms → diagnosis), optimizing for precision and recall.
- API Integration: Deploy your model via a cloud service like AWS SageMaker, exposing it as an API for your app to call.
- User Interface: Design an intuitive UI that collects user inputs (e.g., symptom checklists) and displays results clearly, with actionable next steps.
- Regulatory Compliance: Ensure HIPAA compliance by encrypting user data and implementing secure authentication—non-negotiable for trust.
Leading AI Diagnostic Tools and Vendors
Developers have a growing array of AI diagnostic tools to integrate into apps. Here are key players shaping the market:
- Philips SmartSpeed: Accelerates MRI scans with AI, integrating with hospital apps for faster diagnostics (FDA-approved, 2023).
- IDx-DR: Automates diabetic retinopathy screening, syncing with EHRs for seamless app deployment (FDA-approved, 2018).
- Arterys CardioAI: Uses cloud-based AI for cardiac imaging, reducing analysis time by 40%.
- VUNO Med®-BoneAge: Assesses pediatric bone age via X-rays, enhancing pediatric care apps.
- Prenosis Sepsis ImmunoScore: Predicts sepsis risk, integrated into clinical apps (FDA-approved, 2024).
These tools offer APIs for easy app integration, enabling healthcare mobile app developers to build apps that rival traditional diagnostics. For example, IDx-DR’s API allows apps to screen retinal images in real time, boosting accessibility.
Top Use Cases in Healthcare Apps
Here’s a perfect example showcasing how AI became a lifesaver for a 27-year-old French woman, Marly Garnreiter.
According to a report by People.com, in early 2024, she suffered various symptoms like night sweats and itchy skin. Marly dismissed these symptoms for a while, crediting her stress and grief over her father’s loss.
Even her tests came out normal once she saw a doctor. However, once she shared her symptoms with ChatGPT, it hinted that Marly could have blood cancer.
After dismissing these symptoms for a few months, as suggested by her friends, Marly felt some pain in her chest. She got tested again and had Hodgkin lymphoma, a type of blood cancer.
While ChatGPT is not a diagnostic tool, in this case, it highlighted an overlooked concern that prompted further testing.
And that was a real-world example of what AI can achieve for people’s health. Now, a little bit about other possibilities:
- Chronic Disease Management: Chronic diseases like diabetes are crucial to be managed in real-time. AI gives apps the required power to track it by leveraging the abilities of IoT in healthcare and diabetes apps. Here’s an example: DreaMed Diabetes uses the abilities of AI diagnostics in healthcare to analyze glucose readings and recommend insulin adjustments.
- Mental Health Monitoring: Woebot leverages AI in medical diagnostics to detect signs of anxiety or depression through user interactions, integrating NLP for sentiment analysis.
- Early Cancer Detection: Freenome employs AI for medical diagnosis identification to screen blood for early cancer markers, a feature you can replicate with biomarker data.
- Preventive Care: Binah.ai uses AI in healthcare diagnostics to monitor vitals via smartphone cameras, predicting risks like heart disease.
Future Trends in AI Diagnostic Tools for Apps
The integration of AI, especially in critical medical requirements, is on the rise. For instance, according to The Grand View Research report, the market for AI in cancer diagnosis is rising between 2025 and 2030 at 24.1%. But that’s just one trend, there are plenty others to keep an active eye on if you’re planning to launch a mobile app to target the healthcare industry.
Let’s have a look!
- Market Growth: The market of AI in diagnostics is set to touch $5.24 billion by 2030, compared to $1.75 billion in 2025. That rise is triggered by the fact that AI isn’t only for end-users but also healthcare workers. These folks can keep an eye on patients remotely, inspiring them to integrate AI into more processes to improve the quality of the modern healthcare system.
- AI in medical imaging: The implementation of using AI to gather data using medical imaging and diagnostics is another example of demonstrating critical role of the tech. According to a report by Global Market Insights, 2024, 75.2% of AI-related healthcare applications were focused on using medical imagining to gather data.
- Wearable Integration: The wearable AI market is rising at a CAGR of 17.2% between 2024 and 2029. The tech is already revolutionizing healthcare tracking and experimenting with more advanced features such as predicting possible health issues, personalizing medicines, and helping medical professionals gather more accurate patient data.
- Personalized Medicine: Tempus, a healthtech company, uses AI diagnostics in healthcare to tailor cancer treatments with genetic data, requiring integration with genomic databases.
- Global Accessibility: The World Economic Forum highlights AI’s role in underserved regions, like TB screening in rural areas. You can build apps with offline capabilities to ensure AI in medical diagnostics reaches everyone.
Challenges and Ethical Considerations for Developers
Building apps with AI in healthcare diagnostics comes with challenges:
- Data Privacy: Users fear breaches. Implement end-to-end encryption and HIPAA compliance to ensure trust in AI diagnostics in healthcare. Leverage the advantages of AI in cybersecurity for better digital security of your healthcare solutions.
- Algorithmic Bias: Non-diverse data can lead to misdiagnoses. Use inclusive datasets and explainable AI, like PathAI in pathology, to mitigate bias.
- Scalability: Optimize models for speed with techniques like model pruning, and deploy on scalable cloud platforms like AWS to handle high volumes.
- Accessibility: The World Economic Forum stresses inclusivity. Design apps for low-resource settings with offline modes and multilingual support to broaden the reach of AI in healthcare diagnostics.
Ethically, transparency matters. The National Library of Medicine notes that AI in medical diagnostics must complement human expertise, ensuring doctors remain in the loop. The final diagnosis will only be controlled by medical professionals. Provide clear disclaimers about AI limitations and integrate feedback loops for model improvement.
Development Costs and Considerations
Integrating AI diagnostics into apps requires investment. Healthcare app development costs range from $50,000 to $500,000, depending on:
- Data Processing: Curating datasets like MIMIC-III ($10,000–$50,000).
- Model Development: Training and optimizing models ($20,000–$100,000).
- Compliance: Ensuring HIPAA and FDA compliance ($10,000–$50,000).
- Cloud Infrastructure: Deploying via AWS or Azure ($5,000–$50,000 annually).
Startups can leverage pre-trained models from vendors like Philips or VUNO to reduce app development costs, making AI diagnostics accessible to smaller teams.
Why This Matters for Healthcare App Developers and Tech Professionals
For healthcare app developers and tech professionals, AI in healthcare diagnostics offers a chance to build tools that save lives, like Marly’s cancer detection, or improved global health, as seen in rural TB screenings.
With searches for AI in medical diagnosis witnessing a surge of 85.19% between January and December (Google Trends 2024), the opportunity is clear: experts are exploring ways of leveraging AI diagnostics in healthcare, promising both impact and profitability.
That shows an opportunity, as well as the probability for the future of healthcare diagnostics being shaped by the possibilities of AI. So, it’s time for you to buckle up and grab the opportunity existing in the market, whether you’re building an app yourself or looking forward to hire healthcare app developers.
Frequently Asked Questions
-
How is AI transforming diagnostics in healthcare?
-
How is AI improving cancer diagnostics?
-
What is the future of AI in medical diagnostics?
-
How is artificial intelligence transforming healthcare?

Content Writer
Sakshi Kaushik is a wordsmith extraordinaire who transforms complex technical jargon into captivating, must-read articles. Armed with a Masters in Economics, Sakshi dissects intricate topics with the precision of a seasoned expert. Her insights have graced prestigious platforms like Hackernoon, Ecowiser, and Medium, captivating readers and tech aficionados alike. With a career spanning influential companies like Teleperformance, Finex, and SparxIT Solutions, Sakshi is well-versed in navigating both the keyboard and the boardroom.
In addition to her extensive experience, Sakshi holds HubSpot certifications in Digital Advertising and Content Marketing, and has earned further credentials from UpGrad, Coursera, and Great Learning. Dedicated to sharing her expertise with mobile app developers and tech enthusiasts, Sakshi's passion shines through her writing. When she's not crafting compelling content, she enjoys diving into thrilling novels and exploring diverse worlds.